The laboratory had infrared spectroscopy instruments from FOSS and Bruker. With the assistance of the staff from The Artificial Intelligence Institute at Waikato University, and their open-source machine learning software WEKA, we soon found that we could build much better models using machine learning than we could using the software supplied by the instrument manufacturers.
Model Building As A Craft?
The conventional approach to calibration modelling seemed to be something of a craft, requiring highly skilled 'chemometricians' who applied their knowledge of analytical chemistry to painstakingly develop the perfect model. This approach is admirable but difficult to scale.
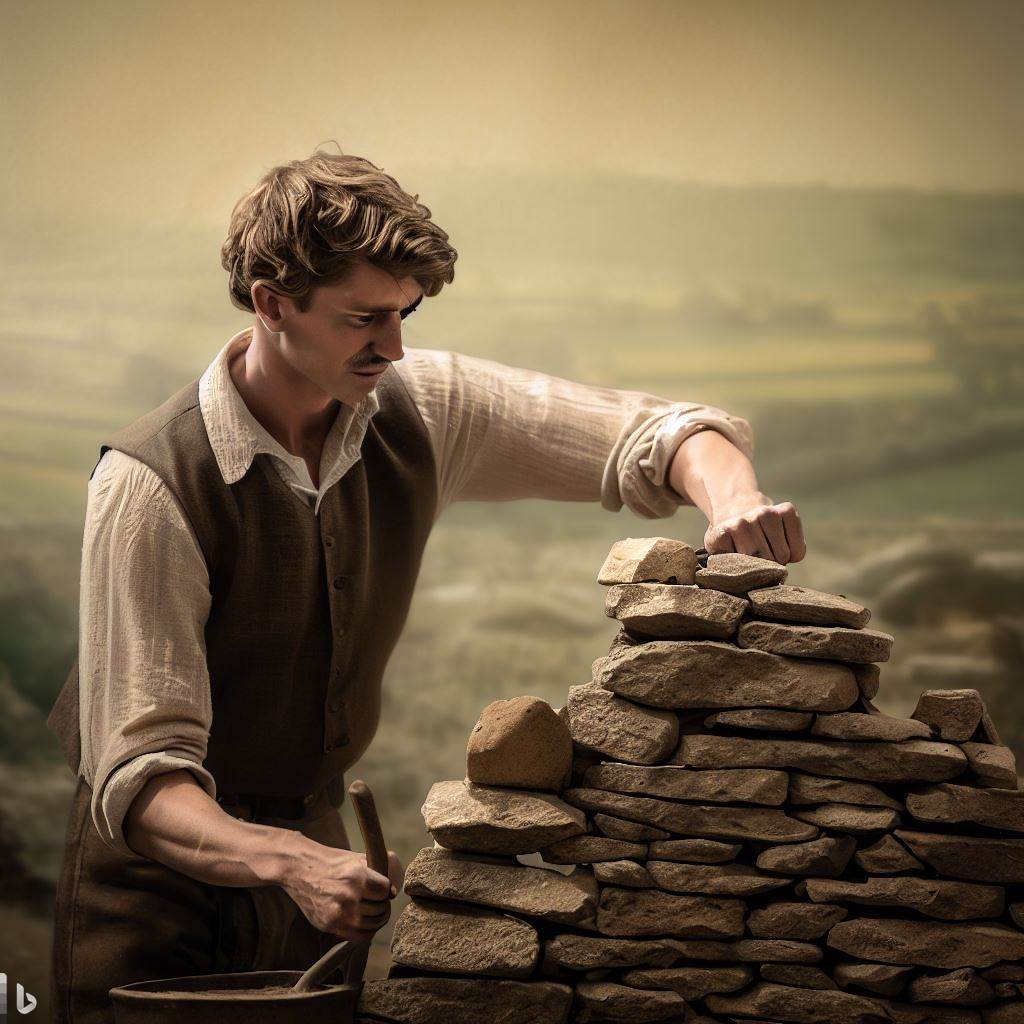
Leave It To The Computer
Sagitto's approach is to try not to second guess the computer. For example, we like to start with the full wavelength range rather than presume to know in advance which parts of the spectrum contain the most important information. And we let the computer decide which algorithms to use, and what parameters to apply to them.
Benchmarking Existing Models
Invariably we find that our machine learning models compare very favourably in performance against calibration models built using software programs like Matlab, or software provided by instrument manufacturers such as Bruker OPUS, Perten PerCal Plus, Agilent Microlab or Buchi NIRCal. If you're curious to see whether your current models could be improved further by applying machine learning to your data, we'd be delighted to benchmark your models against the best that we can produce.
Over the years we've developed very streamlined data handling systems, and the process of building NIR and FTIR calibration models has become highly automated for us. For this reason we're able to offer our benchmarking service free of charge.
Click here to find out more.
Acknowledgements
Special thanks to the following :-
OpenAI DALL-E 2 for bullseye image
Microsoft Bing Image Creator powered by DALL-E for the image of a dry stone wall builder (who for some inexplicable reason seems to have a bucket)